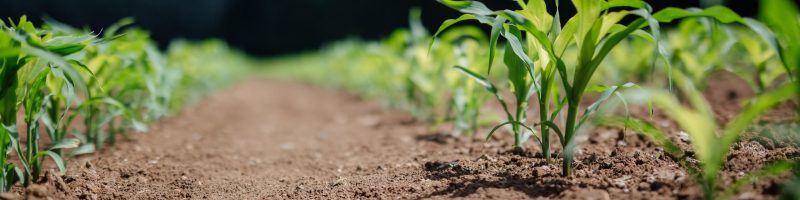
ABSTRACT – Vineyards from hilly areas of the Mediterranean region are mostly grown in shallow soils that present low soil water holding capacity. These vineyards are prone to water stress conditions, which if well managed can raise the grape quality potential. This study aimed to investigate the water stress development in an “Aglianico” vineyard grown along a 90 m slope. The two-year (2011–2012) trial was conducted in two soils having different hydraulic properties, the Up-slope with lower soil water holding capacity than the Down-slope site. The results showed that grapevines were more stressed in the Up-slope soil than in the Down-slope soil, as reflected by the higher crop water stress index, lower leaf water potential and leaf gas exchanges values. Consequently, the yield was significantly lower by 40% in the Up-slope, which was determined by the lower weight and volume of berries. The smaller berries improved must quality parameters of total soluble solids, total polyphenols, total anthocyanins, and color intensity within a range of 4–25% higher in the Up-slope compared to the Down-slope site. Moreover, the pre-veraison stress experienced in 2012 reduced yield by 30% and depressed berry weight and volume, compared to 2011. The post-veraison stress induced the improvement of must quality, mainly in the Up-slope 2011. Interestingly, there was no significant difference in the pH and titratable acidity between both sites, which indicates the ability of Up-slope vines to make up for more stressful conditions, and, thus, their resilient behavior to maintain their high-quality wine. This study highlights that vineyards in hilly areas may benefit from a differentiated management between different viticulture zones to bring up their high-quality wine.
ABSTRACT – Water stress and in particular drought are some of the most significant factors affecting plant growth, food production, and thus food security. Furthermore, the possibility to predict and shape irrigation on real plant demands is priceless. The objective of this study is to characterize, classify, and forecast water stress in tomato plants by means of in vivo real time data obtained through a novel sensor, named bioristor, and of different artificial intelligence models. First of all, we have applied classification models, namely Decision Trees and Random Forest, to try to distinguish four different stress statuses of tomato plants. Then, we have predicted, through the help of recurrent neural networks, the future status of a plant when considering both a binary (water stressed and not water stressed) and a four-status scenario. The obtained results are very good in terms of accuracy, precision, recall, F-measure, and of the resulting confusion matrices, and they suggest that the considered novel data and features coming from the bioristor, together with the used machine and deep learning models, can be successfully applied to real-world on-the-field smart irrigation scenarios in the future.
ABSTRACT – In the framework of the on-going research project E-Crops ( www. e-crops. it/), coordinated by the Institute of Bioeconomy of the Italian National Research Council (NRC), and founded by Italian Ministry of University and Research, several Precision Agriculture (PA) technologies are under development and testing for different agricultural systems and fields of application, at both farm and district scales. All data and information provided by these PA technologies are integrated in a common cloud platform and elaborated by a unique DSS (Decision Support System).
ABSTRACCT – Extracting knowledge from natural images captured in-field requires semantic segmentation to precisely detect fruits or other objects of interest. This paper demonstrates the generalizability of deep networks trained on a highly variable dataset in the context of grape segmentation in vineyards. Among the proposed architectures, the DeepLabv3-ResNext returned the best results, scoring a noticeable 95.1% balanced accuracy during the test. This best model was then transferred to segment images of other datasets, captured by different cameras, and focusing on other grape varieties. Results proved the robustness of the DeepLabv3-ResNext, thus bringing the proposed methodology closer to its actual use.
ABSTRACT – Traditionally, strawberries are harvested manually when the typical colour of the cultivar does not reach at least 80% of the surface. The focus of this research activity is to develop an automatic system based on image analysis in order to objectively define the optimal harvest time. Strawberries (cv. Sabrosa), with different degrees of maturation, were analyzed in four different harvesting periods and subsequently selected and classified, based on the ripening percentage, in three maturity classes: R025, R5070 and R75100. Each class of 10 strawberries, evaluated in triplicate, was subjected to image analysis and physiological and qualitative evaluation by measuring the following parameters: respiratory activity, pH, total soluble solids content, and titratable acidity. The images, captured by a digital camera, were processed using Matlab® software and all the data found were supported by multivariate analysis. The image processing has made it possible to create an algorithm measuring objectively the percentage and the saturation level of red assigning the fruit to each Class. Principal component analysis (PCA) shows that discriminating parameters are the Chroma and the red Area, then used in a Partial LastSquare Regression (PLSR) model to predict the TSS/TA ratio with R2 of 0.7 and 0.6 for calibration and validation set, respectively.
ABSTRAC – This paper describes a novel image acquisition and processing framework to detect and count grape bunches along vineyard rows, using RGB and depth data acquired in the field by a farmer robot. The proposed pipeline starts with a semantic image segmentation module that uses a pre-trained convolutional neural network to separate fruit from non-fruit regions. Areas pertaining to fruits are then further processed using a depth gradient-based clustering algorithm to detect and separate single grape bunches. Experiments performed in a commercial vineyard are presented showing that, despite the low quality of the input images, the proposed approach is able to correctly detect and count grape clusters with good accuracy.
ABSTRACT – Since 2010, more and more farmers have been using remote sensing data from unmanned aerial vehicles, which have a high spatial–temporal resolution, to determine the status of their crops and how their fields change. Imaging sensors, such as multispectral and RGB cameras, are the most widely used tool in vineyards to characterize the vegetative development of the canopy and detect the presence of missing vines along the rows. In this study, the authors propose different approaches to identify and locate each vine within a commercial vineyard using angled RGB images acquired during winter in the dormant period (without canopy leaves), thus minimizing any disturbance to the agronomic practices commonly conducted in the vegetative period. Using a combination of photogrammetric techniques and spatial analysis tools, a workflow was developed to extract each post and vine trunk from a dense point cloud and then assess the number and position of missing vines with high precision. In order to correctly identify the vines and missing vines, the performance of four methods was evaluated, and the best performing one achieved 95.10% precision and 92.72% overall accuracy. The results confirm that the methodology developed represents an effective support in the decision-making processes for the correct management of missing vines, which is essential for preserving a vineyard’s productive capacity and, more importantly, to ensure the farmer’s economic return.
LEGGI L’ARTICOLO
Deep learning methods can provide accurate segmentations for plant monitoring in precision agriculture. However, acquiring and annotating a sufficient number of images to train deep segmentation models is a tedious and time-consuming task. This drawback can be tackled by means of data augmentation methods, and recently mixing methods have achieved good results. In this work, 11 mixing data augmentation methods have been applied to construct segmentation models of natural images of a vineyard. The experiments show that by applying these mixing strategies, the performance of the segmentation models can be 4.90% better than models trained with traditional data augmentation techniques.
ABSTRACT – This study investigates the efficacy of Synthetic Aperture Radar (SAR)-based vegetation indexes for filling gaps in the optical-driven Leaf Area Index (LAI) time series. The statistical properties of coherent (e.g., interferometric coherence) and incoherent (e.g., backscattered signal) SAR vegetation indexes are systematically studied, including their correlation with LAI measurements and significance for LAI reconstruction. First, the Multi-Output Gaussian Process (MOGP) algorithm is selected, analyzed, and subsequently improved to handle the non-Gaussian distribution of the exploited SAR indexes. Hence, a refined MOGP method incorporating a quantile-transform (QT) operation is proposed. Experiments focus on the Arborea zone in Sardinia, Italy, exploiting one year of optical and radar images from the European Copernicus Sentinel-1/2 sensors. The results prove the usefulness of the refined MOGP model in obtaining LAI time series with reduced uncertainties (R2 = 0.9/0.7 training/validation) and filling gaps in optical-based LAI observations, reconstructing feasible crop dynamic along the season. The study also provides insights into phenological state evolution and implications for future applications of the presented method.
ABSTRACT – Automatic yield monitoring and in-field robotic harvesting by low-cost cameras require object detection and segmentation solutions to tackle the poor quality of natural images and the lack of exactly-labeled datasets of consistent sizes. This work proposed the application of deep learning for semantic segmentation of natural images acquired by a low-cost RGB-D camera in a commercial vineyard. Several deep architectures were trained and compared on 85 labeled images. Three semi-supervised learning methods (PseudoLabeling, Distillation and Model Distillation) were proposed to take advantage of 320 non-annotated images. In these experiments, the DeepLabV3+ architecture with a ResNext50 backbone, trained with the set of labeled images, achieved the best overall accuracy of 84.78%. In contrast, the Manet architecture combined with the EfficientnetB3 backbone reached the highest accuracy for the bunch class (85.69%). The application of semi-supervised learning methods boosted the segmentation accuracy between 5.62 and 6.01%, on average. Further discussions are presented to show the effects of a fine-grained manual image annotation on the accuracy of the proposed methods and to compare time requirements.
ABSTRACT – An innovative low-cost device based on hyperspectral spectroscopy in the near infrared (NIR) spectral region is proposed for the non-invasive detection of moldy core (MC) in apples. The system, based on light collection by an integrating sphere, was tested on 70 apples cultivar (cv) Golden Delicious infected by Alternaria alternata, one of the main pathogens responsible for MC disease. Apples were sampled in vertical and horizontal positions during five measurement rounds in 13 days’ time, and 700 spectral signatures were collected. Spectral correlation together with transmittance temporal patterns and ANOVA showed that the spectral region from 863.38 to 877.69 nm was most linked to MC presence. Then, two binary classification models based on Artificial Neural Network Pattern Recognition (ANN-AP) and Bagging Classifier (BC) with decision trees were developed, revealing a better detection capability by ANN-AP, especially in the early stage of infection, where the predictive accuracy was 100% at round 1 and 97.15% at round 2. In subsequent rounds, the classification results were similar in ANN-AP and BC models. The system proposed surpassed previous MC detection methods, needing only one measurement per fruit, while further research is needed to extend it to different cultivars or fruits.
ABSTRACT – The widespread agricultural use of the phenylurea herbicide Diuron (DCMU) requires the investigation of ecotoxicological risk in freshwater and soil ecosystems in light of potential effects on non-target primary producers and a heavier effect on higher trophic levels. We used microalgae-based fluorimetric bioassays for studying the interferences on the photosynthesis of a freshwater and soil model green microalga (Chlamydomonas reinhardtii) induced by environmentally relevant concentrations of the herbicide DCMU. Measurements of steady-state chlorophyll a (Chl-a) fluorescence emission spectra were performed; as well, the kinetics of the Chl-a fluorescence transient were recorded. Percentage indexes of interference on photosynthesis were calculated after comparison of steady-state and kinetic Chl-a fluorescence measurements of DCMU-exposed and control C. reinhardtii cell suspensions. The results obtained after 30 min exposure to the herbicide DCMU confirmed a significant inhibitory effect of DCMU 2 μg/L, and no significant differences between %ι values for DCMU 0.2 μg/L and 0.02 μg/L exposures. Positive %ε values from kinetic measurements of the Chl-a fluorescence transient confirmed the same interfering effect of 2 μg/L DCMU on PSII photochemistry in the exposed C. reinhardtii cell suspensions. Negative values of %ε observed for 0.2 and 0.02 μg/L DCMU exposures could be attributable to a presumptive ‘stimulatory-like’ effect in the photochemistry of photosynthesis. Short-term exposure to sub-μg/L DCMU concentration (≤0.2 μg/L) affects the photosynthetic process of the model microalga C. reinhardtii. Similar environmental exposures could affect natural communities of unicellular autotrophs, with hardly predictable cascading secondary effects on higher trophic levels.
ABSTRACT – Vineyard classification is an important process within viticulture-related decision-support systems. Indeed, it improves grapevine vegetation detection, enabling both the assessment of vineyard vegetative properties and the optimization of in-field management tasks. Aerial data acquired by sensors coupled to unmanned aerial vehicles (UAVs) may be used to achieve it. Flight campaigns were conducted to acquire both RGB and multispectral data from three vineyards located in Portugal and in Italy. Red, green, blue and near infrared orthorectified mosaics resulted from the photogrammetric processing of the acquired data. They were then used to calculate RGB and multispectral vegetation indices, as well as a crop surface model (CSM). Three different supervised machine learning (ML) approaches—support vector machine (SVM), random forest (RF) and artificial neural network (ANN)—were trained to classify elements present within each vineyard into one of four classes: grapevine, shadow, soil and other vegetation. The trained models were then used to classify vineyards objects, generated from an object-based image analysis (OBIA) approach, into the four classes. Classification outcomes were compared with an automatic point-cloud classification approach and threshold-based approaches. Results shown that ANN provided a better overall classification performance, regardless of the type of features used. Features based on RGB data showed better performance than the ones based only on multispectral data. However, a higher performance was achieved when using features from both sensors. The methods presented in this study that resort to data acquired from different sensors are suitable to be used in the vineyard classification process. Furthermore, they also may be applied in other land use classification scenarios.
ABSTRACT – Olea europaea L. is a glycophyte representing one of the most important plants in the Mediterranean area, both from an economic and agricultural point of view. Its adaptability to different environmental conditions enables its cultivation in numerous agricultural scenarios, even on marginal areas, characterized by soils unsuitable for other crops. Salt stress represents one current major threats to crop production, including olive tree. In order to overcome this constraint, several cultivars have been evaluated over the years using biochemical and physiological methods to select the most suitable ones for cultivation in harsh environments. Thus the development of novel methodologies have provided useful tools for evaluating the adaptive capacity of cultivars, among which the evaluation of the plant-microbiota ratio, which is important for the maintenance of plant homeostasis. In the present study, four olive tree cultivars (two traditional and two for intensive cultivation) were subjected to saline stress using two concentrations of salt, 100 mM and 200 mM. The effects of stress on diverse cultivars were assessed by using biochemical analyses (i.e., proline, carotenoid and chlorophyll content), showing a cultivar-dependent response. Additionally, the olive tree response to stress was correlated with the leaf endophytic bacterial community. Results of the metabarcoding analyses showed a significant shift in the resident microbiome for plants subjected to moderate salt stress, which did not occur under extreme salt-stress conditions. In the whole, these results showed that the integration of stress markers and endophytic community represents a suitable approach to evaluate the adaptation of cultivars to environmental stresses.
ABSTRACT – Automated in-field data gathering is essential for crop monitoring and management and for precision farming treatments. To this end, consumer-grade digital cameras have been shown to offer a flexible and affordable sensing solution. This paper describes the integration and development of a cost-effective multi-view RGB-D device for sensing and modeling of agricultural environments. The system features three RGB-D sensors, arranged to cover a horizontal field of view of about 130 deg in front of the vehicle, and a suite of localization sensors consisting of a tracking camera, an RTK-GPS sensor and an IMU device. The system is intended to be mounted on-board an agricultural vehicle to provide multi-channel information of the surveyed scene including color, infrared and depth images, which are then combined with localization data to build a multi-view 3D geo-referenced map of the traversed crop. The experimental demonstrator of the multi-sensor system is presented along with the steps for the integration of the different sensor data into a unique multi-view map. Results of field experiments conducted in a commercial vineyard are included, as well, showing the effectiveness of the proposed system. The resulting map could be useful for precision agriculture applications, including crop health monitoring, and to support autonomous driving.
ABSTRACT – Volatile compounds, quality traits (total phenols and antioxidant capacity) and High-performance liquid chromatography (HPLC)-isolated polyphenols of strawberries, variety Sabrosa, commercially referred to as “Candonga”, harvested at three different times (H1, H2 and H3) and at two different ripening stages, namely half-red (Half-red-H1, Half-red-H2 and Half-red-H3) and red (Red-H1, Red-H2 and Red-H3) were evaluated. Dominant anthocyanins, namely cyanidin-3-O-glucoside, pelargonidin-3-O-glucoside and pelargonidin-3-O-rutinoside, as well as p-coumaryl hexoside increased during harvesting, differently from flavonoids, such as quercetin-3-O-glucoside, kaempferol-3-O-glucoronide and quercetin 3-O-glucoronide, that declined. Samples clustered in different quadrants of the principal component analysis (PCA) performed on volatiles, quality traits and phenolic compounds, highlighting that only the red samples were directly correlated to volatile components, as volatiles clearly increased both in number and amount during ripening. In particular, volatiles with a positive impact on the consumers’ acceptance, including butyl butyrate, ethyl hexanoate, hexyl acetate, nonanal, terpenes and lactones, were positively associated with the Red-H1 and Red-H2 strawberries, while volatiles with negative coefficients related to consumer liking, including isopropyl butyrate, isoamyl butyrate and mesifurane directly correlated with the Red-H3 samples. Accordingly, strawberries harvested at Red-H1 and Red-H2 ripening stages could be preferred by the consumers compared to the Red-H3 fruit. Altogether, these results could help to individuate quality traits as putative markers of the ripening stage, and optimize the process of post-harvesting ripening to preserve or improve the desirable aromatic characteristics of strawberries.
ABSTRACT – Despite a drought- and erosion-tolerant root system, olive trees are vulnerable to abiotic stress due to limited genetic variability. Though some olive cultivars are moderately tolerant to salinity stress, soil salinity is increasing in the semi-arid and arid regions where olive cultivation is common, significantly reducing overall production. In response, breeding programs may rely on proper selection markers for abiotic stresses, including salinity, but these are generally lacking for olive. Here, physiological and biochemical parameters were measured in four Olea europaea genotypes (Frantoio, Leccino, Lecciana, and Oliana) subjected to different intensities of salinity stress (0 mM, 100 mM and 200 mM NaCl). At moderate and high salt concentrations, Na+ exclusion, higher photosynthetic productivity and tissue water content in the tolerant cultivar Frantoio were linked with increased production of polyphenols, with more favorable K+/Na+values (quercetin and rutin), mitigation of oxidative stress (oleuropein) and increased water absorption (luteolin). In Frantoio and Leccino, a significant change of the proteome repertoire occurred, with overrepresentation of components regulating cellular metabolism, ion transport, redox insult and dissipation of excess photochemical energy. Conversely, Lecciana and Oliana showed increased sensitivity to salinity stress in terms of photosynthetic parameters and elevated internal Na+ concentrations, together with the lowest number of differentially represented proteins. These results highlighted olive germplasm strategies to cope with osmotic stress, suggested a physiological and molecular basis for the augmented responsiveness of tolerant cultivars and identified specific biomarkers as useful targets for future breeding programs.